Caltech/INQNET hosts Quantum Transduction workshop
9/21/2018

Researchers gathered on September 21 to discuss the state-of-the-art, challenges, directions, news ideas and research towards quantum transduction for applications in various areas including quantum networks. The Quantum Transduction workshop hosted at Caltech as part of the Caltech INQNET research program was chaired by Calgary’s Christoph Simon and Caltech/INQNET’s Maria Spiropulu. Emphasis in the discussion was placed on quantum microwave-to-optical transduction and associated technologies. Such transduction methods are essential for the realization of a long-distance quantum network that will allow for an efficient interface between many types of stationary qubits and flying qubits. Approaches that were discussed during the workshop included opto-mechanical systems, electro-optical systems, and atomic ensembles among others. Motivated by many fruitful discussions at the workshop we plan to compose an overview paper detailing the state-of-the art and novel directions of this critical emerging field. Carl Willimas of NIST, gave a keynote address and discussed federal plans at DOE, NIST and NSF among other agencies and offices, and coordination with OSTP on a new Quantum Science Initiative to bolster Quantum Science and Technology Research in the US. The link to the workshop presentations can be found here: https://indico.hep.caltech.edu/event/583/.
For further information, contact Maria Spiropulu ms2piATcaltech.edu
INQNET/FQNET at “Next steps in Quantum Science for HEP” at Fermilab
9/12/2018
Neil Sinclair of INQNET/Caltech presented the status of the Fermilab quantum teleportation experiments (FQNET) at the “Next steps in Quantum Science for HEP” workshop organized at Fermilab. This is the first presentation of the experimental setup and commissioning results of the FQNET system that was installed earlier in the year at Fermilab. The workshop focused on applications of quantum computing technologies, algorithms, and theoretical developments of interest to the High Energy Physics community. The Fermilab Quantum Network aims to produce a fully functional quantum network initially based on the distribution of time-binned photonic quantum entanglement. The resulting quantum network system will serve fundamental research and future R&D on complex quantum communication technologies and protocols. The workshop presentations, including the FQNET one, can be found at https://indico.fnal.gov/event/17199/.
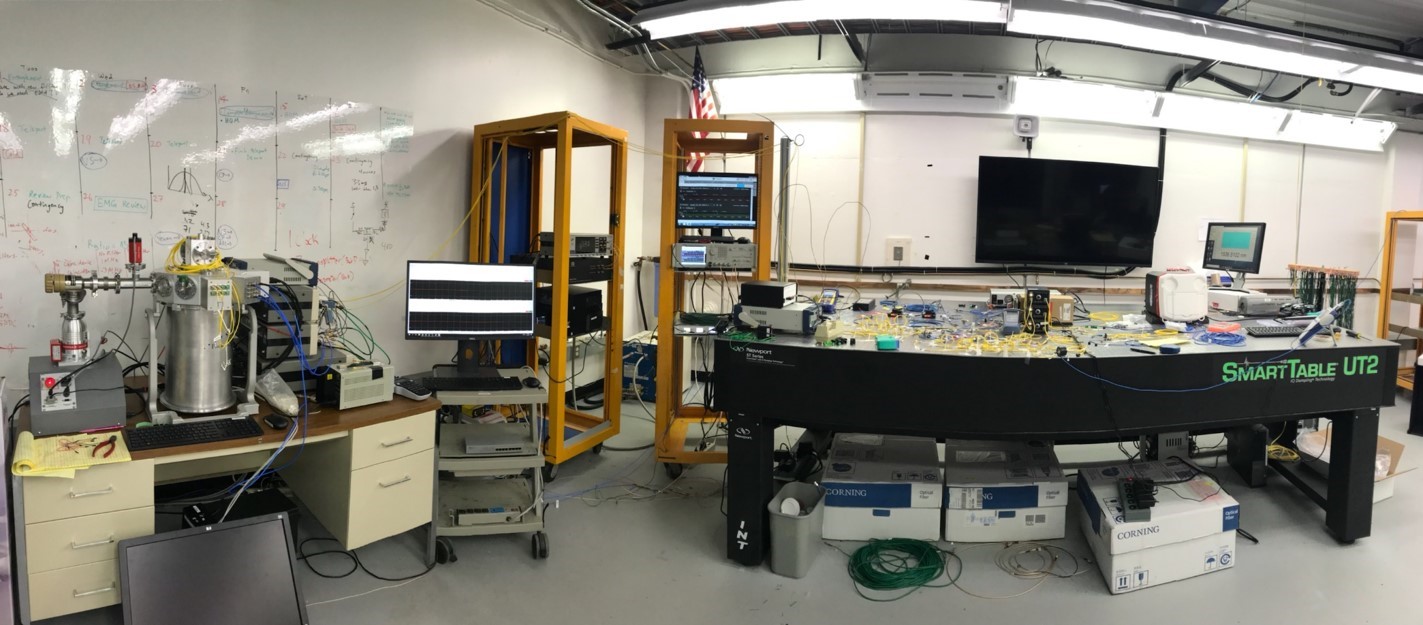
For further information, contact Maria Spiropulu ms2piATcaltech.edu
Futurecast: The Future of Quantum Technologies
8/24/2018
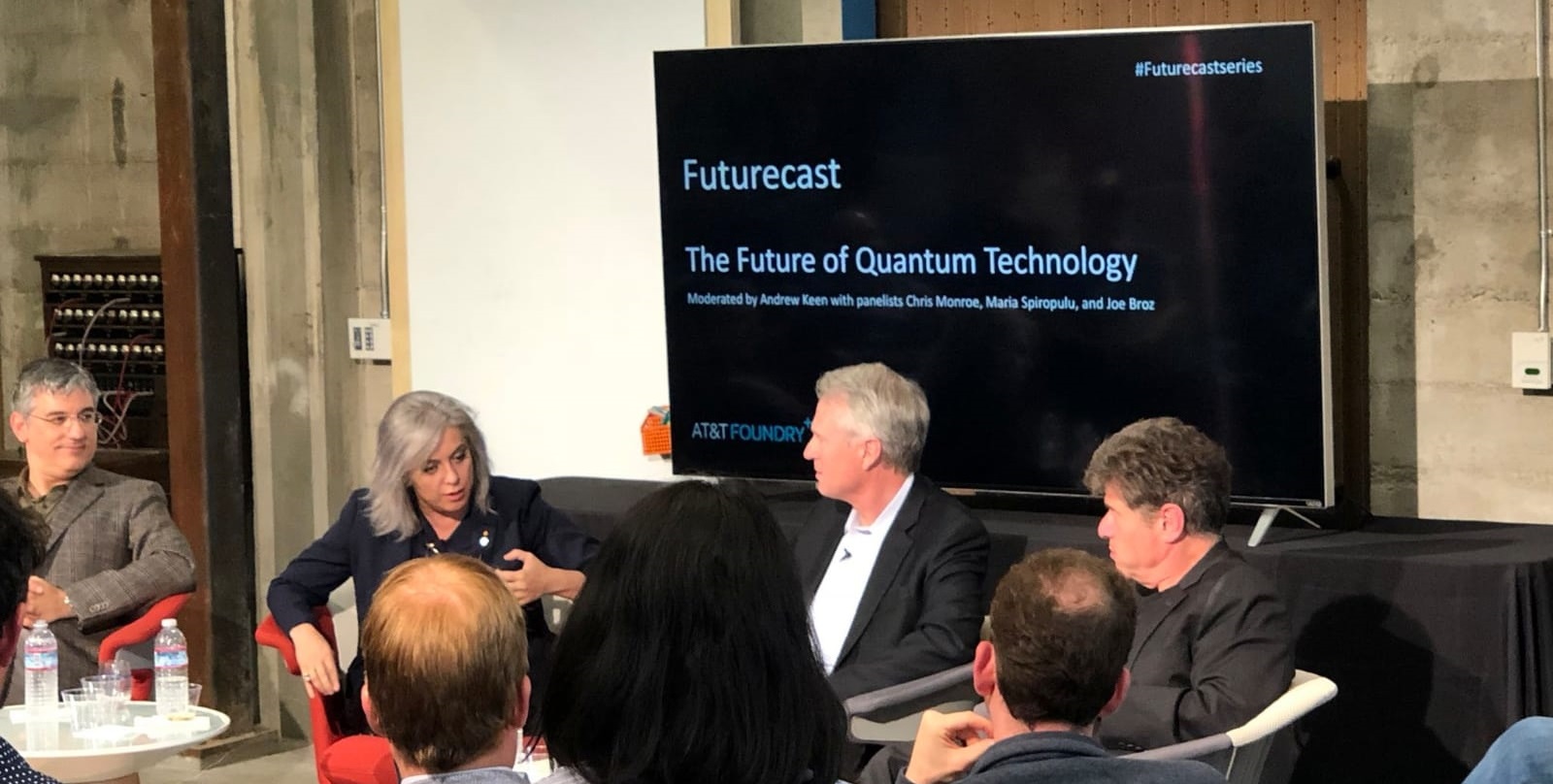
Join the Intelligent Quantum Networks and Technologies (INQNET) program, the AT&T Foundry and Ericsson for the upcoming Futurecast event “The Future of Quantum Technologies” on August 24, 2018. Futurecast is a thought leader series designed to vet, debate, and ultimately spark ideas that that will set the course of our collective technological future. In this Futurecast, we will explore the current state of quantum technologies. Generally regarded as a class of devices that actively create, manipulate and read out quantum states of matter using the quantum effects of superposition and entanglement, quantum technologies are no longer a thing of the future.
Moderated by Andrew Keen, this panel discussion will look to discuss the following questions: What is the roadmap to quantum computers? What is the level of coordination in academia, government, and the private sector today? How can we increase coordination and collaboration? Should we, as a society, take a step back or should we be accelerating?
Join INQNET at the AT&T Foundry as we speak with leading experts Chris Monroe, Maria Spiropulu, and Joe Broz to explore the future of quantum technology.
About The Principal Guests
![]() |
Joseph Broz
A physicist by training, Joseph Broz, Ph.D, leads SRI’s Advanced Technology and Systems Division (ATSD) in Applied Science, government business development and technical and business strategy. He brings 35 years of experience in both the public and private sectors to this role—including an extensive background in corporate business development and deep subject matter expertise in solid state physics, quantum systems, electromagnetics, micromagnetics, nuclear magnetic resonance, countering weapons of mass destruction and the C4ISR mission spaces. Broz has worked closely with governors, national laboratories, and he has significant experience with projects for the Department of Defense and the Department of Homeland Security. |
![]() |
Maria Spiropulu
Maria Spiropulu is Shang-Yi Ch'en Professor of Physics at Caltech. Her experimental research includes the Higgs boson, dark matter, supersymmetry, and the origins of space-time. She is founder of the Physics of the Universe Summit and founder/PI of the Alliance for Quantum Technologies targeting quantum networks & communications, and quantum AI. |
![]() |
Christopher Monroe
Christopher Monroe is a leading quantum information scientist and atomic/optical physicist, and is a Distinguished University Professor of Physics at the University of Maryland and Joint Quantum Institute. He demonstrated the first multiqubit quantum gate realized in any system, and more recently has demonstrated new ways to scale atomic qubits and simplify their control with semiconductor chip traps, pulsed lasers, and photonic interfaces for long-distance entanglement. He is co-founder and chief scientist at IonQ in College Park, MD, a company that fabricates complete-stack quantum computers based on trapped atomic ions. |
Content provided by AT&T Foundry
For further information, contact Maria Spiropulu ms2piATcaltech.edu
Caltech and AT&T present Quantum Networks R&D program at SuperComputing17 in Denver
11/14/2017
The Alliance for Quantum Technologies (AQT), founded by Caltech and AT&T in May 2017 in collaboration with national laboratories and industry partners, is presenting the “INtelligent Quantum NEtworks & Technologies” (INQNET) research program at Supercomputing 2017 in Denver (November 13-16). “The consortium will accelerate progress in quantum science and technologies by bringing together the strengths of government, academia, and industry in a basic science R&D framework,” says Shang-Yi Ch’en Professor of Physics Maria Spiropulu of Caltech.
The INQNET program currently focuses on quantum networks and quantum machine learning. “Quantum networks promise to provide not only ultra-secure communication channels, but could prove critical to developing scalable quantum computing infrastructure,” says, Melissa Arnoldi, president of AT&T Technology Operations.
To this end, the Fermilab quantum network teleportation experiment (FQNET) is being built as part of the INQNET R&D program. “FQNET represents a basic step towards improved entanglement-based networks,” says Cristián Peña, the co-spokesperson of the FQNET experiment and a Lederman Fellow at Fermilab. “With NASA’s Caltech-managed Jet Propulsion Laboratory, we are developing sensors to improve the efficiency and reliability of the entanglement distribution.”
“We are looking forward to testing these technologies in the industry setting,” says Yewon Gim, a senior member of the technical staff of the AT&T Foundry innovation center and an INQNET fellow. “At Fermilab with FQNET we’re trying to push the next phase of instrumentation.”
The INQNET quantum networks group expects the first phase of the FQNET project to produce results by late spring. “The plan is to build eventually a prototype quantum internet, first with nodes in the Chicago area and then around the U.S.,” says Si Xie, a Caltech postdoctoral scholar based at Fermilab who works on the Large Hadron Collider physics and detector upgrades research program.
“We like the model for INQNET,” says Joseph Lykken, the deputy director of Fermliab. “This is a model we know moves very fast. Labs are good for doing things on a larger scale. Industry can bring resources quickly. This can be a very nimble and flexible program.”
“We’re doing a lot of community building,” says Soren Telfer, the director of the Palo Alto AT&T Foundry innovation center. “I already had three interns over the summer who did projects on quantum networks and quantum machine learning.”
“INQNET interns both at graduate and undergraduate level are engaging on quantum technology projects and are rapidly very productive and extremely creative,” says Rishi Pravahan, senior scientist at AT&T, who spearheads the INQNET program at the Foundry.
“With the infusion of expertise from AT&T, Caltech, Fermilab, and others, we are taking a fresh approach in developing quantum networks. We expect that our results will push forward quantum technology while at the same time addressing the greatest fundamental questions in physics,” says Neil Sinclair, an award-winning expert on long-distance quantum technologies who has accepted an offer to join INQNET as a fellow with Harvard and Caltech.
Beyond the near-term demonstrator and benchmarking projects that could have an impact on industry early adoption, the INQNET program also supports a number of long-term projects tackling fundamental challenges in quantum science. According to Lykken and Xie, the INQNET quantum networks group is looking into the possibility of using the FQNET infrastructure to investigate the famous ER=EPR conjecture, which offers a theoretical bridge between the existence of wormholes between a pair of black holes and quantum entanglement.
Visit the AQT/INQNET Supercomputing17 booths (#663 and #763) at the Denver convention center Nov 13-Nov 16 2017 and experience the quantum teleportation VR demo developed by NovaVR’s Oliver Lykken.
Written by Mark H. Kim
For further information, contact Maria Spiropulu ms2piATcaltech.edu
Solving a Higgs optimization problem with quantum annealing for machine learning
10/18/2017
Machine learning methods are quickly becoming indispensable in experimental high energy physics, with the Higgs discovery example being a notable use-case where machine learning accelerated the discovery by improving the selection of the Higgs decays in many final states particles, especially electrons and photons which are notoriously plagued with ``fakes'', namely backgrounds that mimic the signal.
The LHC general purpose experiments (ATLAS and CMS) were built to probe the mechanisms of electroweak symmetry breaking and the particle origins of dark matter. Wired up with about a hundred million readout channels each and made up of many thousands of tons of material that interacts with the particles emanating from the LHC’s high-energy proton–proton collisions, the two detectors have already in 2012 managed to capture and reconstruct many rare Higgs boson candidate events. They announced the discovery of the Higgs boson the same year and the discovery resulted in the Nobel prize in Physics being awarded to Higgs and Englert in 2013. The Higgs bosons decay into other particles after about 100 yoctoseconds (10-22) and the collider searches involve several different decay signatures or “channels”. One of them involves the Higgs decaying to two photons.
In this work we apply quantum optimization, for the first time, to a Higgs selection problem, via machine learning implemented on an experimental quantum annealing device with more than 1000 qubits. More specifically, our work shows for the first time how to apply, in practice, quantum annealing for machine learning (QAML). Namely we show how to construct weak classifiers and tie them to physics observables used in the discovery of the Higgs boson. We show that these classifiers are highly resilient against overtraining and MC simulation limitations. In addition the method provides intrinsically a way of picking out the most important variables from a larger set of input variables that are informative in terms of their physics content and interpretation.
Importantly, and also for the first time, we go beyond ground state-based classifiers and we exploit excited states to improve, at negligible algorithmic cost, the performance of our classifier. We focus our studies on performance measures related to classification accuracy measured in terms of receiver operator characteristics. This is a novel perspective that allows us to explore quantum annealers beyond the usual speedup studies and exploit the expected differences in sample distributions between quantum annealers and classical samplers.
In this work we uncover that QAML has a an advantage when learning from small datasets. We plan to explore this further by embedding other high energy physics problems involving real-time decision making and data certification with small datasets, as well as problems dealing with precision measurements where clear interpretability is necessary and systematic errors and correlations are crucial for the discovery of very subtle and rare physics effects. We also envision that quantum and simulated annealing solutions can be used as an initialization and booster to machine learning solutions running on classical machines.
We expect that as more domain science problems are attempted in this architecture and its successors, much more will be learned in terms of the applicability and advantages offered by quantum annealing in hard optimization problems in science and beyond. We are confident that this work will inspire new applications in other domains, whether scientific or commercial, as other quantum annealing, analog quantum computing and other quantum computing testbeds are quickly being developed.
The methods used in this work are generalizable to problems of similar difficulty on this and other quantum annealing architectures.
FAQ
Q: Why did we pick the specific Higgs optimization problem
The particular Higgs optimization application was chosen because it is simple, with few kinematic variables needed to fully describe the diphoton system. In large measure, this was so that we could test the performance of quantum annealing, as a-priori we did not know what the relative performance would be, and the largest number of variables we could test on the DW2X was only 36. Armed with the results we report here, the community can move on to test moderately larger problems on the newest version of the D-Wave processor, the D-Wave 2000Q (in which 60-variable fully connected problems can be embedded). Using simulated annealing one can also analyze the performance of the algorithm on far larger problems, possibly with dozens of base kinematic variables and thus hundreds or thousands of variables in the Ising problem, which are infeasible to test on any near-term quantum hardware.
Q: What is the goal of this work? usually we hear that these studies are performed to demonstrate “quantum speedup
Given the size of the optimization problem at hand we did not consider speedup as metric for quantumness. Our scope was to formulate a robust, simple, interpretable technique for solving this classification problem which is highly amenable to implementation on quantum annealers, should future generations become large enough, with dense enough connectivity, and with low enough noise rates to achieve advantages over classical solvers.
Since by leaving out a speedup comparison, quantum annealing and simulated annealing would perform identically if both worked perfectly and found the ground state each time, we introduced the idea of constructing classifiers from excited states, knowing that due to sampling differences quantum annealing and simulated annealing would not end up with identical sets of excited states. We wanted to know whether this difference would result in different classification performance. While we cannot claim a statistically significant difference, it is nevertheless noteworthy that at smallest training sizes we did find a slight growing advantage for DW2X over simulated annealing when accounting for an increasing number of excited states, as seen in Fig.5(c) of the paper.
We stress that the technique is easily interpretable and the role of quantum annealing is that of a subroutine for sampling the Ising problem that may in the future have advantages over classical samplers, either when used directly or as a method of seeding classical solvers with-high quality initial states.
Q: Where is the D-Wave machine used in this work
The USC-Lockheed Martin Quantum Computing Center (QCC) based at the USC Information Sciences Institute (ISI) is the longest running quantum computing center in the world. The QCC was launched in November 2011 with a 128-qubit D-Wave One, upgraded in March 2013 with the 512-qubit D-Wave Two, and again in March 2016 with a 1000-qubit D-Wave 2X. The numbers of operational qubits are: 108, 509, 1098, respectively.
The D-Wave 2X Quantum Computing System
We used the current installation at the QCC which is a third-generation D-Wave 2X chip designed to contain up to 1152 superconducting flux qubits, arranged in a two-dimensional 12x12 array of 144 unit cells of 8 qubits each. Within each cell, each qubit is connected to four others, in a K4,4 bipartite graph arrangement. Depending on whether the cell is in a corner or not, the qubits in each cell make 8 or 16 connections to the qubits in neighboring cells. At the time of this work 1098 of the 1152 qubits were functional (95% yield). The D-Wave 2X supports a minimum annealing time of 5µs.
Q: Is the D-Wave a quantum computer?
The D-Wave is not a general purpose digital quantum computer. It is rather an analog quantum computer and specifically a quantum annealer. Both the quantumness and speedup in these devices are intensely scrutinized topics of ongoing research. The D-Wave company implemented the annealer as proprietary scaled-up architecture of flux qubits . Others in the tech industry and academic research are followig-up with research on open quantum computing testbeds and architectures. Beyond the theoretical arguments and demonstrations/papers from the company, we expect the research community in academia and labs to tackle such architectures and produce results on both the instrumentation and the problems encoded and solved in such machines.
Useful Reading
Quantum algorithm for solving linear systems of equations, by A. Harrow, A. Hassidim, and S. Lloyd.Quantum Machine Learning Algorithms: Read the Fine Print, by Scott Aaronson
Why now is the right time to study quantum computing, by Aram Harrow
Written by M. Spiropulu
For further information, contact Maria Spiropulu ms2piATcaltech.edu
Solving A Physics Optimization Problem with Physics-based Computation
10/18/2017
"Solving a Higgs optimization problem with quantum annealing for machine learning" is the title of our article that will appear in the October 19 Issue of the Journal Nature.
Form science to business, researchers face a large number of planning and optimization tasks, where they have to choose between different options. By assigning numerical measurements to these options, they can pick the best one that suits their needs. Such a process is called optimization. Decision-making optimization problems are among the hardest and researchers are constantly working to find more efficient ways of solving them, including quantum computing. Annealing -- quantum or simulated-- provides a physics-based technique of arriving to solutions of hard optimization problems.
An example optimization problem in high-energy physics arises in the search for the Higgs boson, which was discovered with the Large Hadron Collider (LHC), the largest particle collider in the world. The LHC is built to enhance our understanding of the fundamental forces of nature, including the mechanism that elementary particles get their mass. The LHC detectors boast about a hundred million "readout channels that collect extremely large amounts of data from the particle collisions. They were employed to chase after the Higgs boson, leading to its discovery in 2012 and to the 2013 Nobel prize in Physics.
The Higgs bosons decay into other particles extremely fast, after about 100 yoctoseconds (10-22 seconds), which made the Higgs search extremely challenging. The researchers seek different decay signatures of the Higgs particle in the data. For example, one signature involves the Higgs boson decaying to two photons. In this work we tackle the optimization problem of maximizing accuracy at classifying events generating two photons in the high energy proton-proton collisions as either true Higgs decays ("our signal") or other, non-Higgs standard model processes ("the background"). Using the physics properties of the photons involved we designed machine-learning classifiers capable of running both on quantum annealers and their simulated counterparts on classical computers.
We have implemented the quantum annealing-based classifiers on the USC 1098 qubit D-Wave machine, a quantum annealer capable of solving certain optimization problems. The D-Wave machine is not a general-purpose quantum computer capable of running common software. Building such a machine (a so-called universal digital quantum computer) is a topic of active research.
We demonstrate in this work that our quantum and simulated annealing classifiers are highly resilient to overtraining (a situation when the classifiers describe the training data tightly but cannot model well the independent test data) and to errors due to inaccurate high-order variable correlations in the relevant Monte Carlo simulations. Additionally the quantum annealing methods pick out the most important variables from a larger set of input variables that are informative in terms of their physics content and interpretation. Our studies reveal a hint that the physics-based classifiers (both quantum and classical annealing) outperform the standard machine learning methods for small training data sets.
This work has already stimulated additional studies applying the new physics-based computation methods to problems in high energy physics and computational biology.
Written by M. Spiropulu
For further information, contact Maria Spiropulu ms2piATcaltech.edu